Customer lifetime value (CLV) is a key metric for every customer-centric marketer. Predicting the future value of a customer more accurately and reliably, would help to increase the marketing efficiency. Thus, its importance is widely acknowledged in industry and academia. But surveys show that CLV measurement is often a challenge in business practice. Retailers or other non-contractual businesses often fail to accurately predict customer purchase behavior. In particular, in the medium and long term.
Two reasons are key for this: (1) In non-contractual settings, customers do not formally announce when they will stop purchasing from a business. This contrasts contractual business settings such as insurance firms and poses a great challenge for modeling the future purchase behavior of customers. (2) Further, many businesses are subject to seasonal patterns. They experience for example a summer dip as many regular customers are on holidays or they observe significant increases related to special shopping events like Black Friday or Christmas.
The novel approach accounts for all these challenges and provides accurate and reliable estimates of the future value for each individual customer. Recent benchmarks also show that this probability-based approach not only provides better and more reliable prediction accuracy than machine learning approaches but is also faster to compute and does not require specialized computing infrastructure. By providing an accompanying open-source software, it is readily applicable for any business.
The novelty of the proposed approach is to include previously neglected, but from a practical point of view crucial, context variables in the statistical modeling. For the first time, it is possible to consider the effects of customer characteristics such as income and place of residence as well as dynamic effects such as shopping events. Although this seems logical and perhaps even overdue from a content point of view, the challenge lay in the statistical complexity that such an undertaking entails.
To guarantee reliability of the results across a wide variety of scenarios in corporate practice, this study considered data from three different industries. In addition, several alternative statistical models were calculated as benchmarks, this included widely used „rules of thumb“, established traditional statistical models and latest machine learning approaches. Furthermore, short-, medium-, and long-term forecast periods were considered to account for the widely varying planning horizons in practice. The added value of the proposed novel approach could thus be demonstrated over a broad database. The exemplary performance is shown in Figure 1.
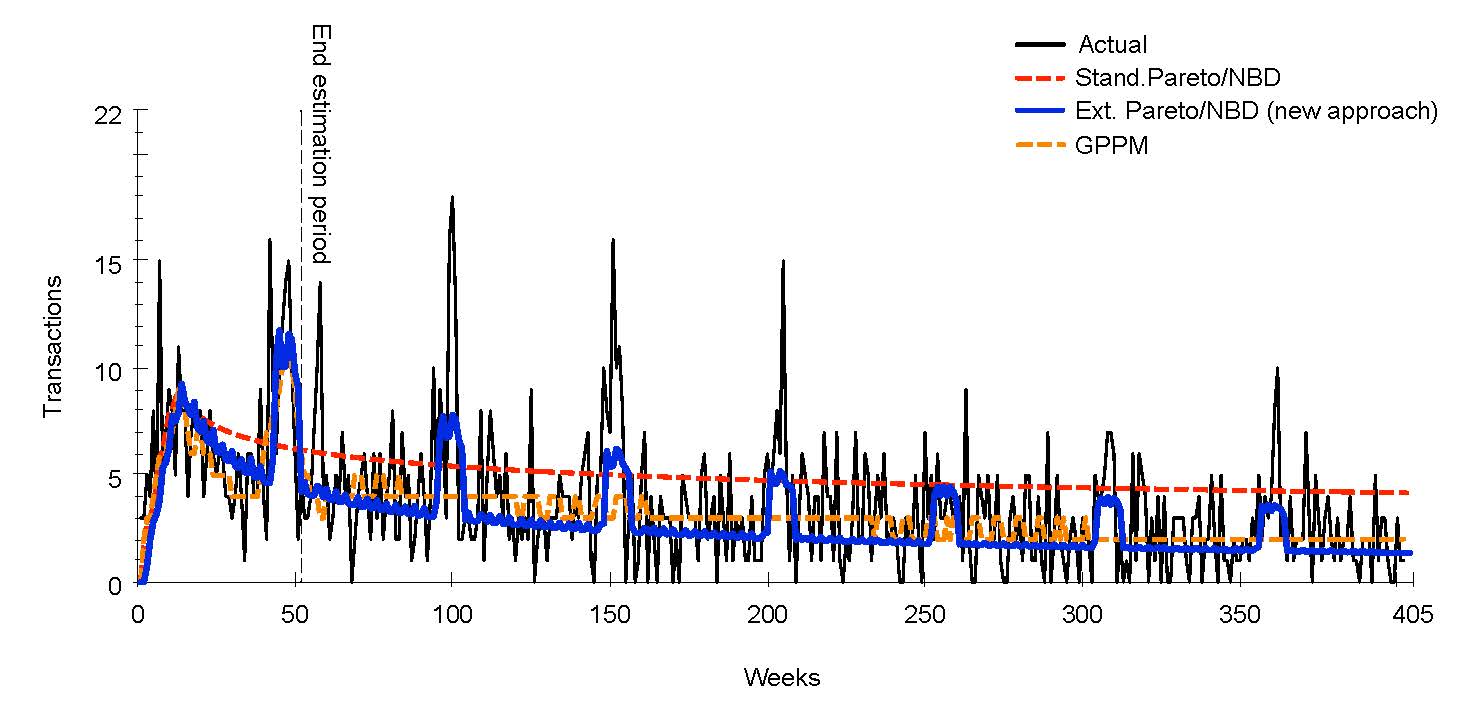
How to use this novel approach as a data analyst
To make the application of this novel approach as easy as possible in practice, an open-source software called „CLVTools“ was developed (www.clvtools.com). CLVTools is a software package for the statistical programming environment R. Beyond the latest work presented here, CVLTools also includes implementations of further complementary and alternative modeling techniques to predict CLV. To date, this software has been downloaded more than 30,000 times.
In its simplest form, without considering any contextual factors, the estimation of CLV only requires a minimal amount of data. At its core, three input variables of customers purchase history are required. This data is available for any firm: a customer identifier (Id), the purchase date, and the purchase amount. Exemplary input data is shown in Table 1.
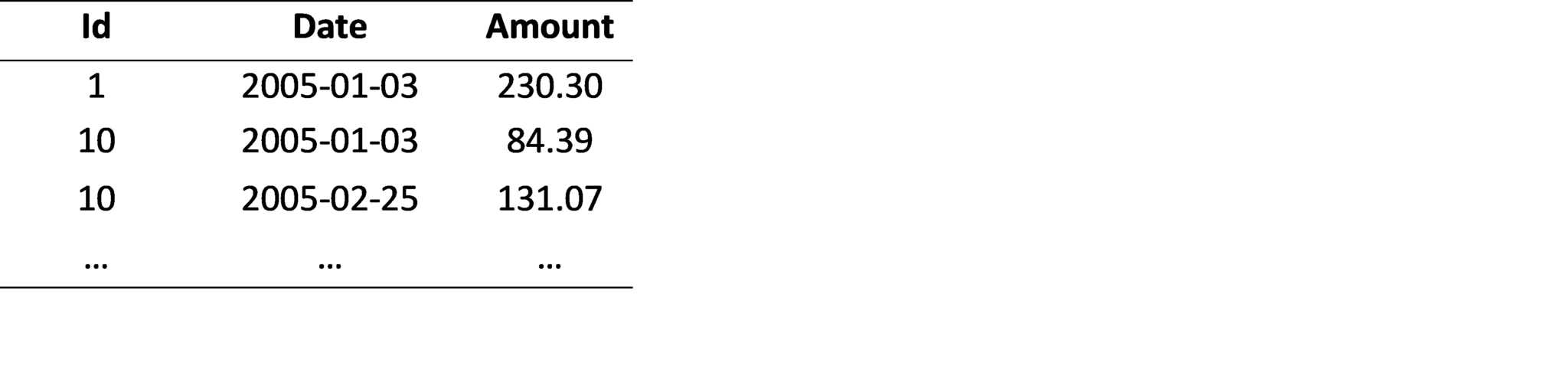
Using the CLVTools package in R only three steps are required to predict customer lifetime value in simplest application case:
• Step 1: Import the historical customer purchase data.
• Step 2: Estimate the model parameters.
• Step 3: Predict the customer purchase behavior.
Figure 2 summarizes the workflow and avai-lable options of CLVTools.
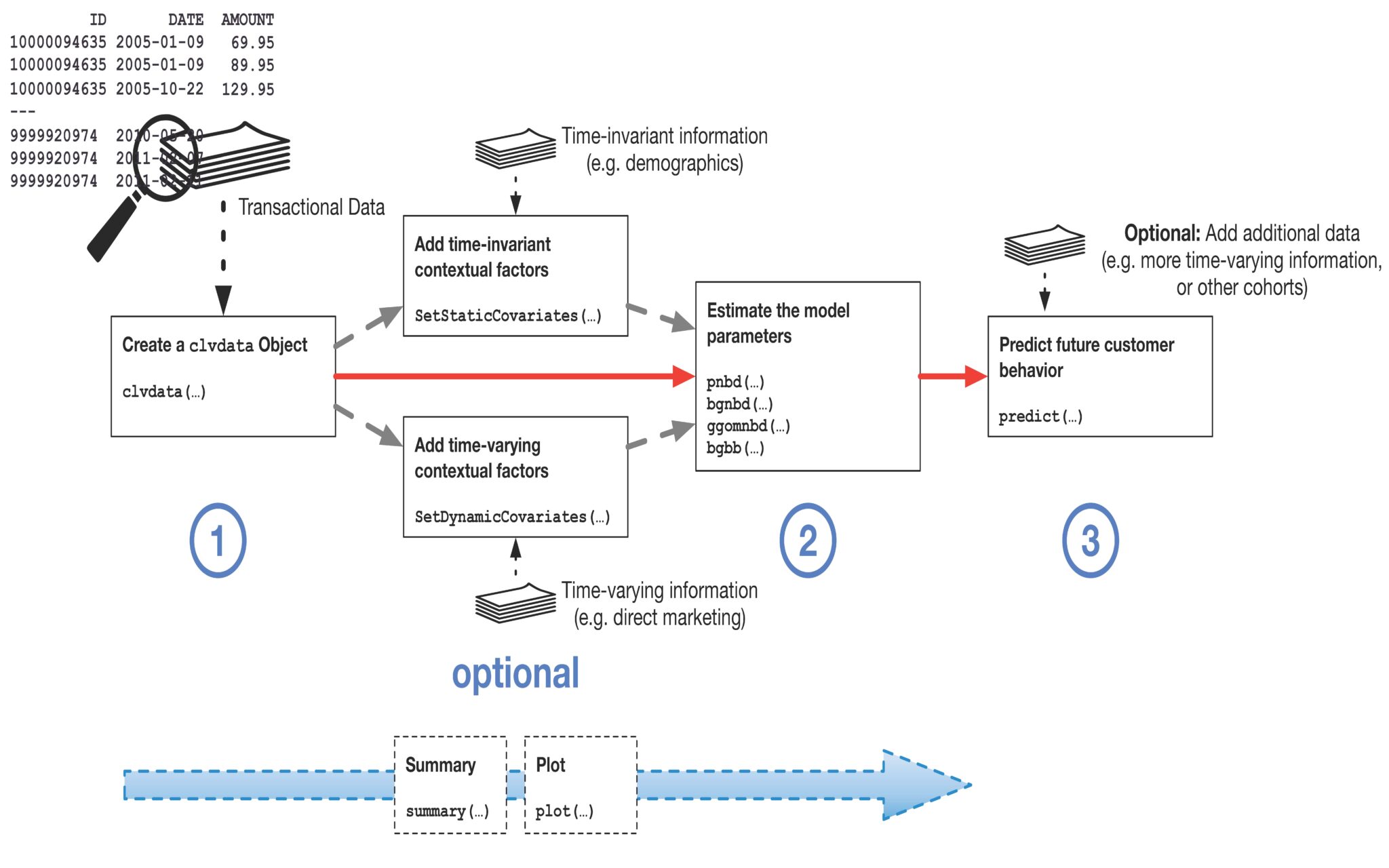
Multiple options to check and plot the data along this workflow are provided. Moreover, advanced model features and alternative model options are available. See https://www.clvtools.com/articles/CLVTools.html for a detailed walkthrough.
Marketing practice thus has a new, freely available tool at its disposal to better coordinate customer-centric marketing activities. Customer value as a central marketing metric is used in a variety of ways, e.g., to individualize coupons, to determine the most effective marketing channels or to allocate resources for win-back campaigns. An accurate calculation of the customer value based on our proposed approach helps to significantly improve the customer-specific personalization and thus the marketing efficiency.
Research Team
Patrick Bachmann (ETH Zurich)
Markus Meierer (University of Geneva)
Jeffrey Näf (ETH Zurich)
Patrik Schilter (Crealytics GmbH)
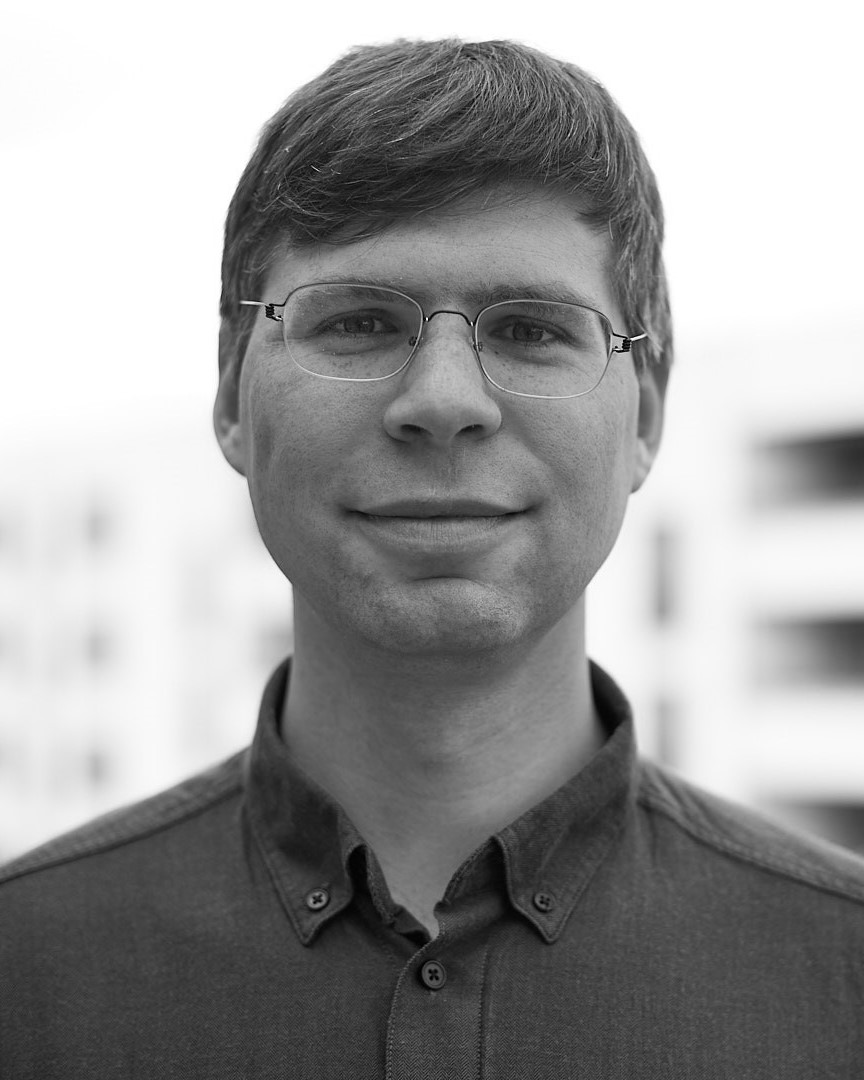
Patrick Bachmann
Post Doctoral Researcher
ETH Zurich
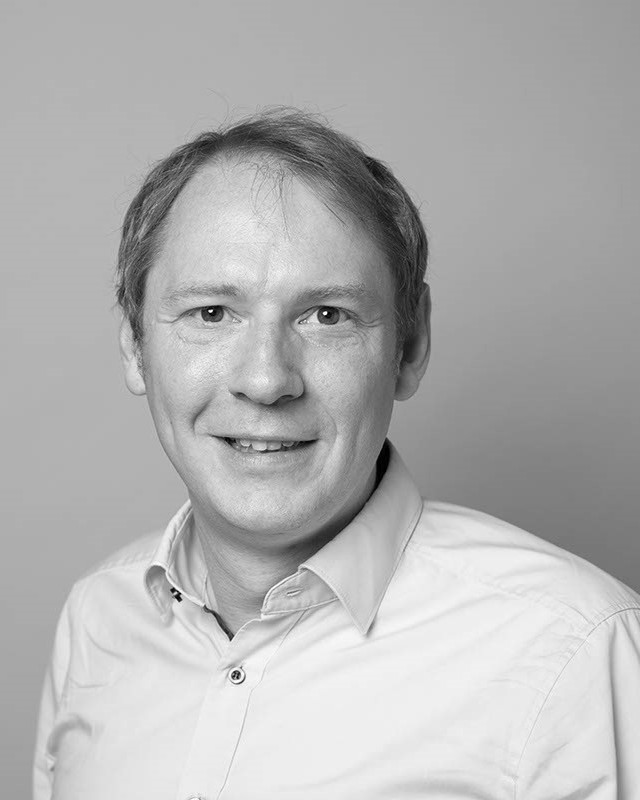
Markus Meierer
Assistant Professor of Marketing
Analytics
University of Geneva
Download Artikel
Swiss Insights News #08
Alle SWISS INSIGHTS News finden Sie hier: SWISS INSIGHTS NEWS